Wide use of AI models in industrial applications
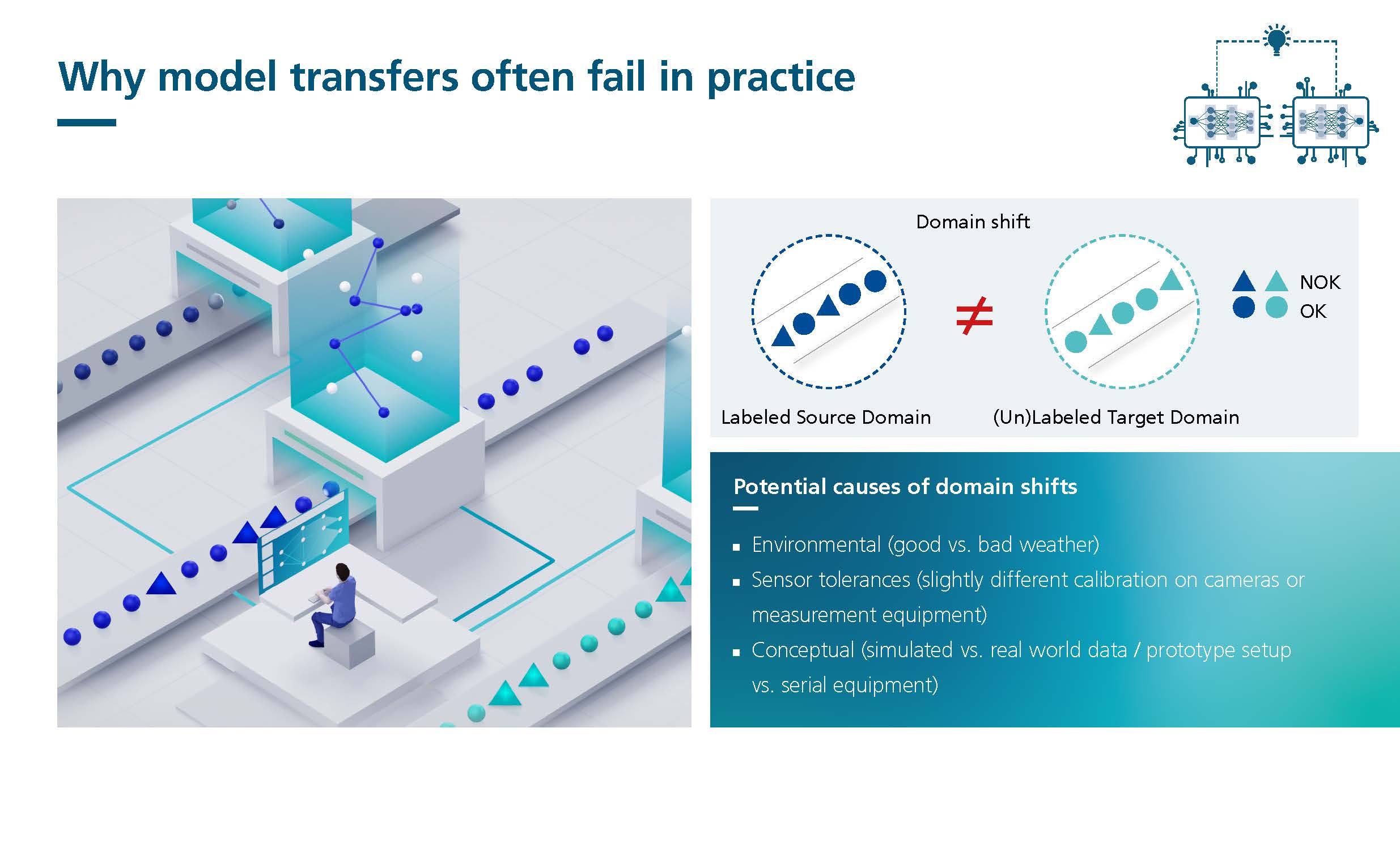
The use of AI models in industry opens up a wide range of possibilities for automating and improving processes. In the case of similar tasks, different production sites, the use of simulated data for real tasks or the adaptation to new conditions, the transfer of machine learning models is particularly useful and can thus save important resources.
However, domain shift means a challenge in the transfer. This term describes the change in the statistical distribution of the data to which the model is applied compared to the training data. Such shifts can have a significant impact on the accuracy of the model results.
Successful use of AI models in industry
To make the use of AI models successful in industry, the following information should be considered:
Task similarity: it is important to check whether the task to be solved in industry is similar to the one for which the model was developed. The greater the similarity, the more likely the model will be successfully transferred.
Production sites: If the model is to be used in different production sites, possible differences in data and environmental conditions must be taken into account. It may be necessary to adapt the model to the specific conditions of each production site in order to achieve optimal results.
Simulated data: The use of simulated data for real tasks can be beneficial. However, it must be ensured that the simulated domain sufficiently represents the real domain. Domain mismatches between simulated and real data can affect the transferability of the model.
Adaptation to new circumstances: When data distributions change, for example due to new circumstances in the industry, the model needs to be adapted. Continuous monitoring of model performance and regular adjustments to new data are crucial here.
Through careful consideration of this information and targeted adaptation of the AI model, the use and also the transfer of AI models in industry can be successfully realised. For this purpose, we use different approaches of transfer learning, e.g. domain adaptation, to adapt existing models well to the conditions described above in a data-efficient way with little additional data of the new domain. The integration of AI technologies enables a more efficient and precise implementation of processes, which can lead to an increase in productivity and quality in industrial applications.