Machine learning and questions of »how« and »why«
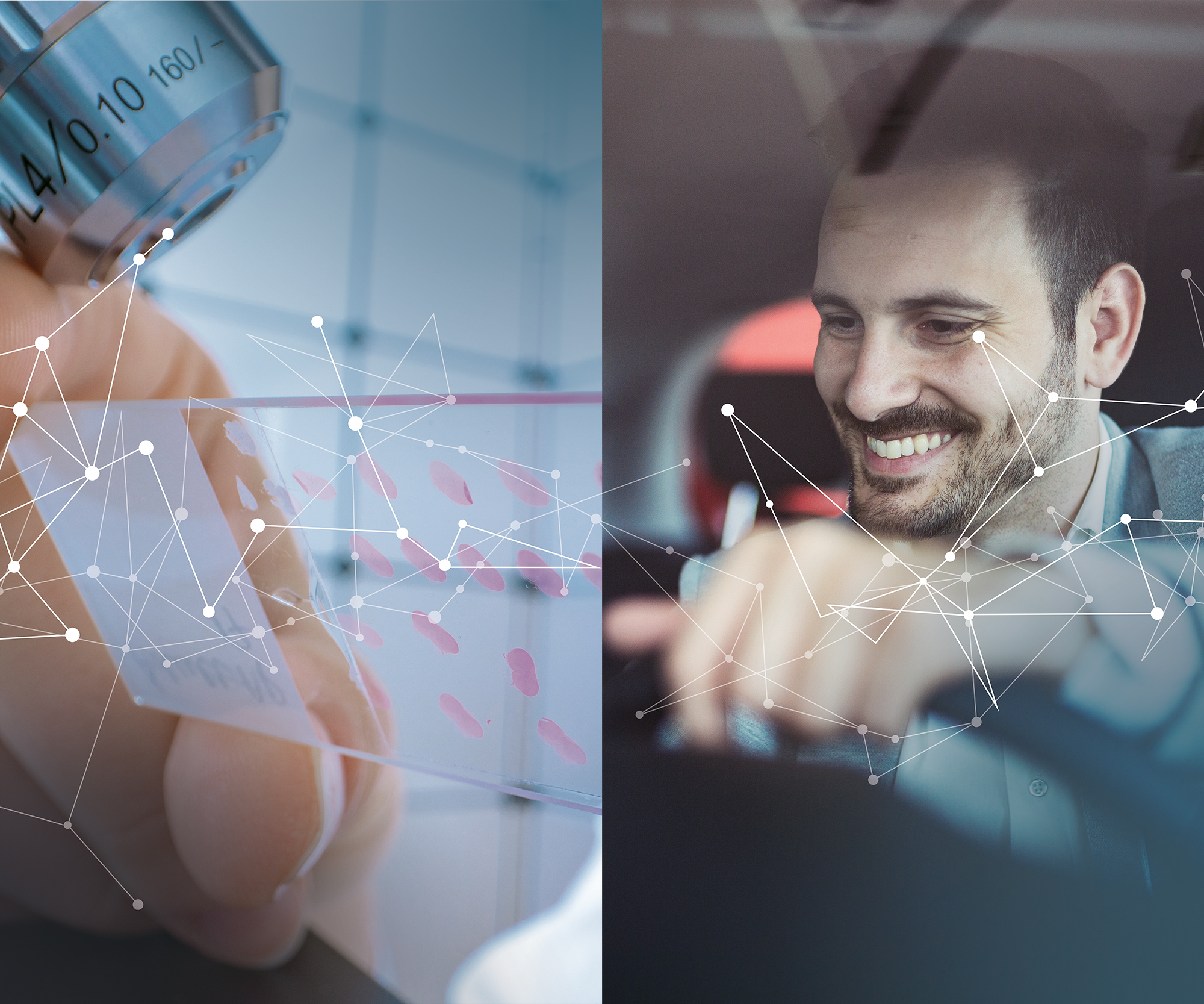
When it comes to the subject of machine learning and deep neural networks (DNNs), there are usually more questions than answers, as data analysis by ML-based systems remains an enigmatic process for developers and users alike. Nevertheless, it is vital that the systems provide transparency and interpretability – particularly with regard to safety issues in the automotive sector, such as driver drowsiness detection, or in medicine, with automated screening of tissue samples.
The use of automatic processes in critical strategic decision-making is dependent on the explainability of data analysis, which also underpins the general acceptance of these processes.