Possibilities for reducing energy consumption
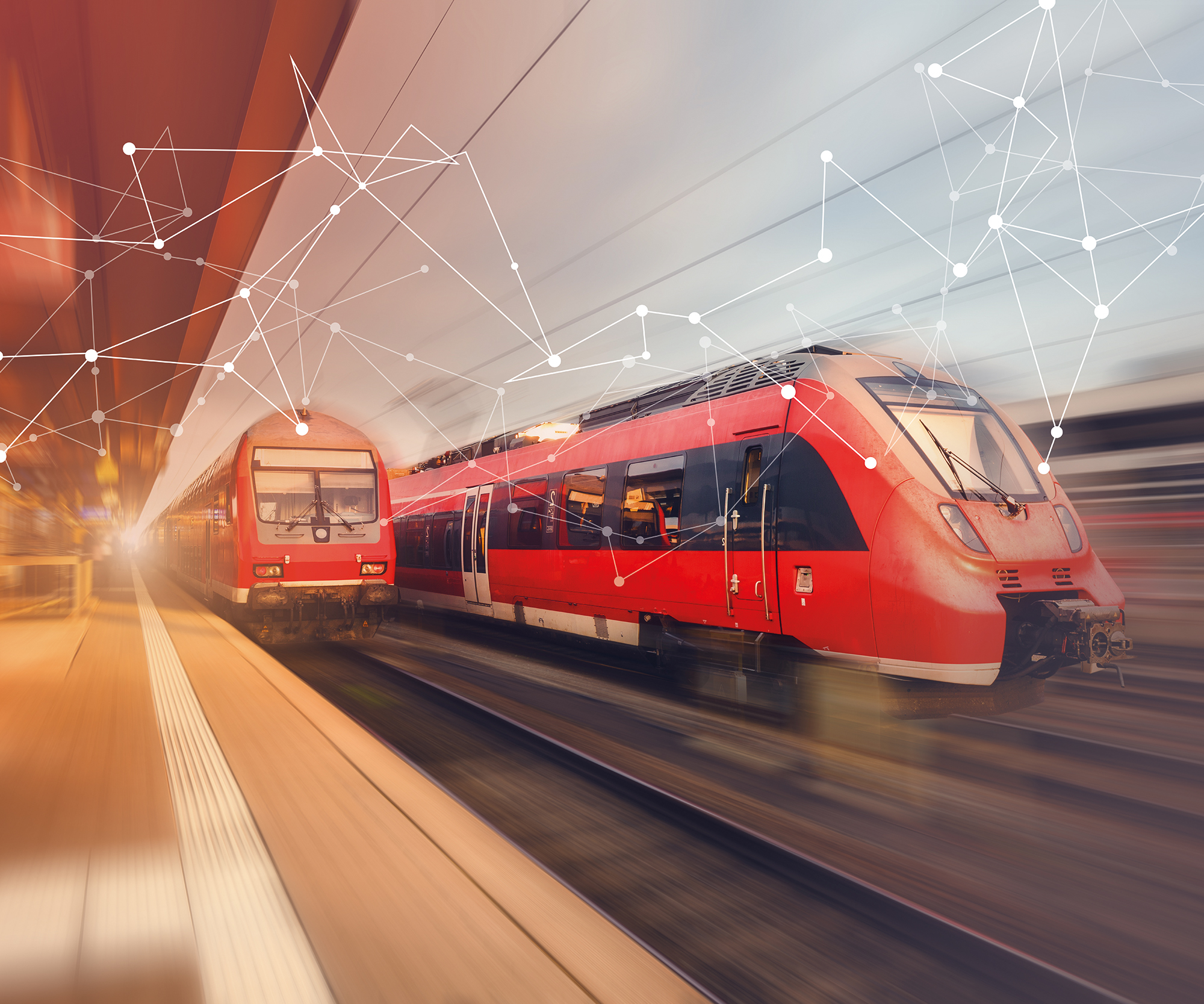
Traction energy consumption is the primary cost factor in the electricity bills of rail transport companies, and is largely determined by how the trains are driven. Energy-efficient speed profiles can therefore lead to significant reductions in power consumption. This includes making the greatest possible use of coasting phases, during which the train consumes no energy. However, it is also vital to coordinate rail traffic with an eye to energy efficiency – for instance by avoiding excessive numbers of simultaneous departures, which result in high peak loads on the electricity grid and therefore additional charges. Moreover, it is important to synchronize arriving and departing trains so that energy recovered while one train is braking can be used to accelerate another.