Automatic generation of parts lists
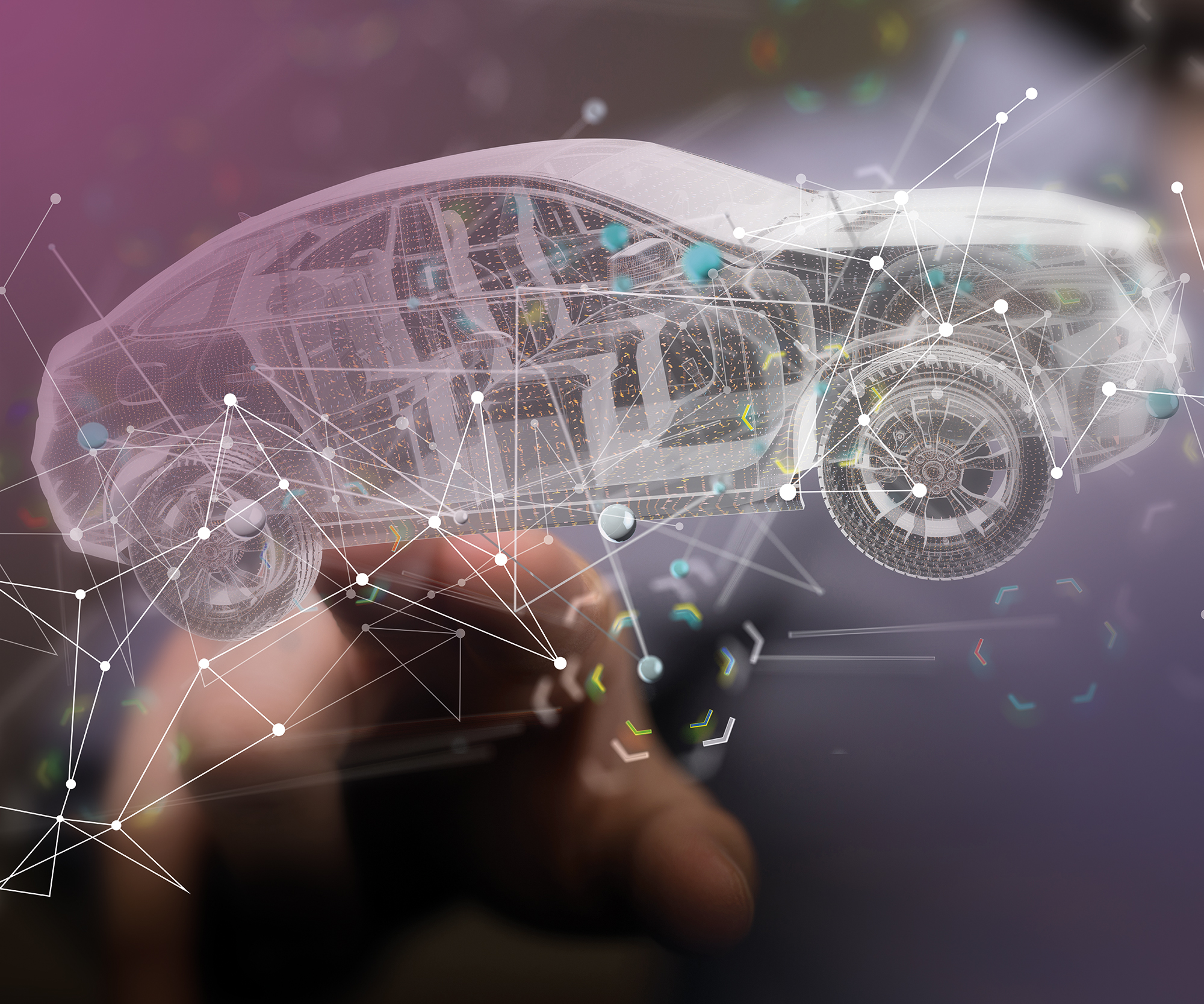
When it comes to preparing an inventory of entire vehicles, the automatic segmentation of three-dimensional datasets from X-ray computed tomography (CT) remains an unresolved challenge. Classical methods are unable to separate different parts and components into voxels and identify them with sufficient reliability. At present, this virtual »dismantling« process can only be performed manually, which is enormously expensive and time-consuming for the industry. There is, however, a great deal of interest on the part of industry, and strong demand for corresponding CT measurements there is an urgent need for solutions that can automatically break the data down into subgroups and convert the resulting volume images of individual assemblies into CAD-compatible formats.