Machine learning in safety-critical systems
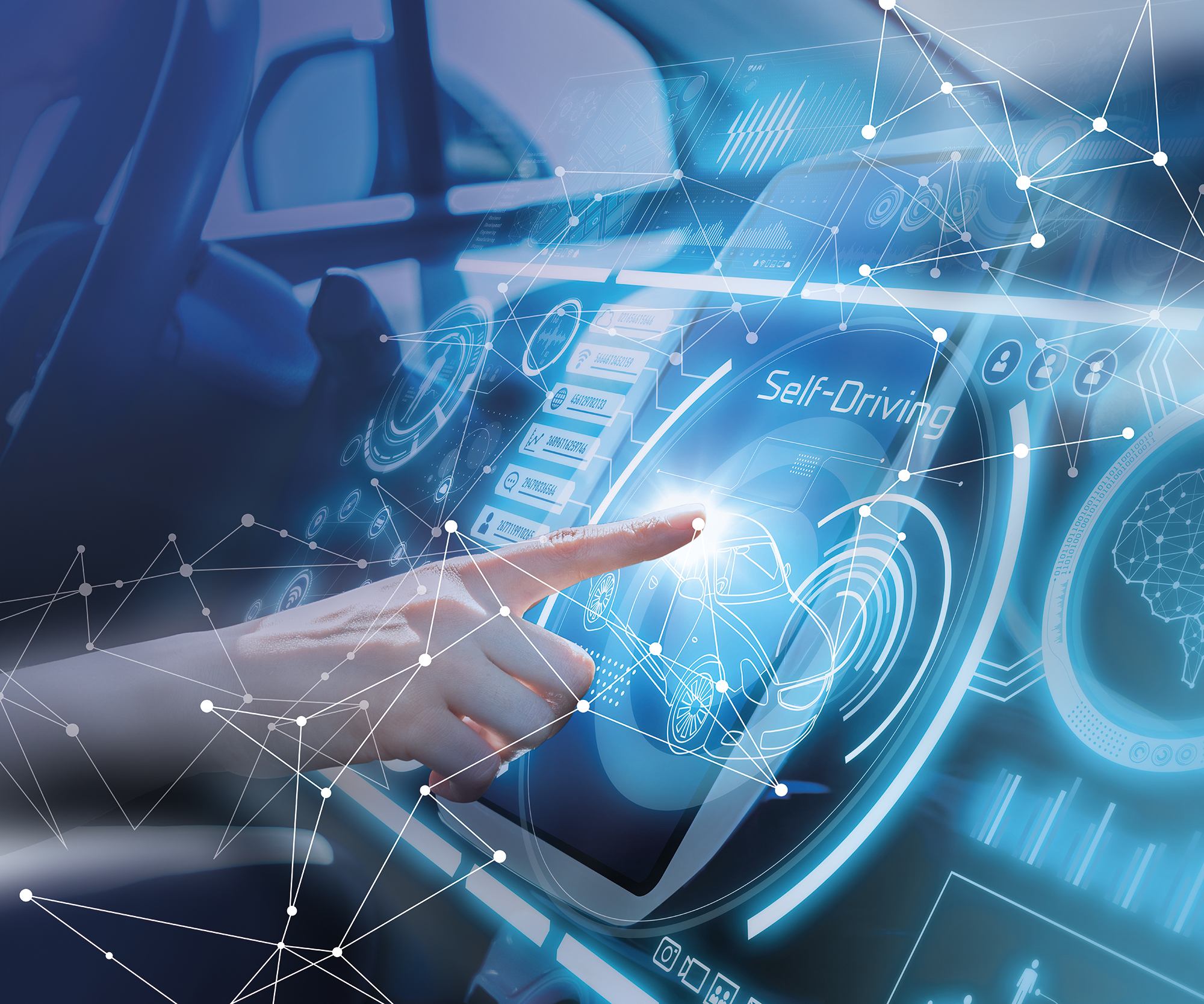
As progressive automation brings us closer to autonomous systems, machine learning methods for mapping and processing complex and unknown situations have become indispensable. Autonomous systems with an advanced degree of automation – i.e., highly automatic to fully autonomous systems – often employ neural networks for context recognition. Early results in the field of deep learning appear highly promising for this task, enabling driverless vehicles to recognize objects, interpret traffic events and issue driving instructions. These techniques, which are based on machine learning, can either be used system-wide, that is from the sensor to the actuator, or merely to solve individual aspects of autonomous driving.