Multimodal detection of cognitive overload
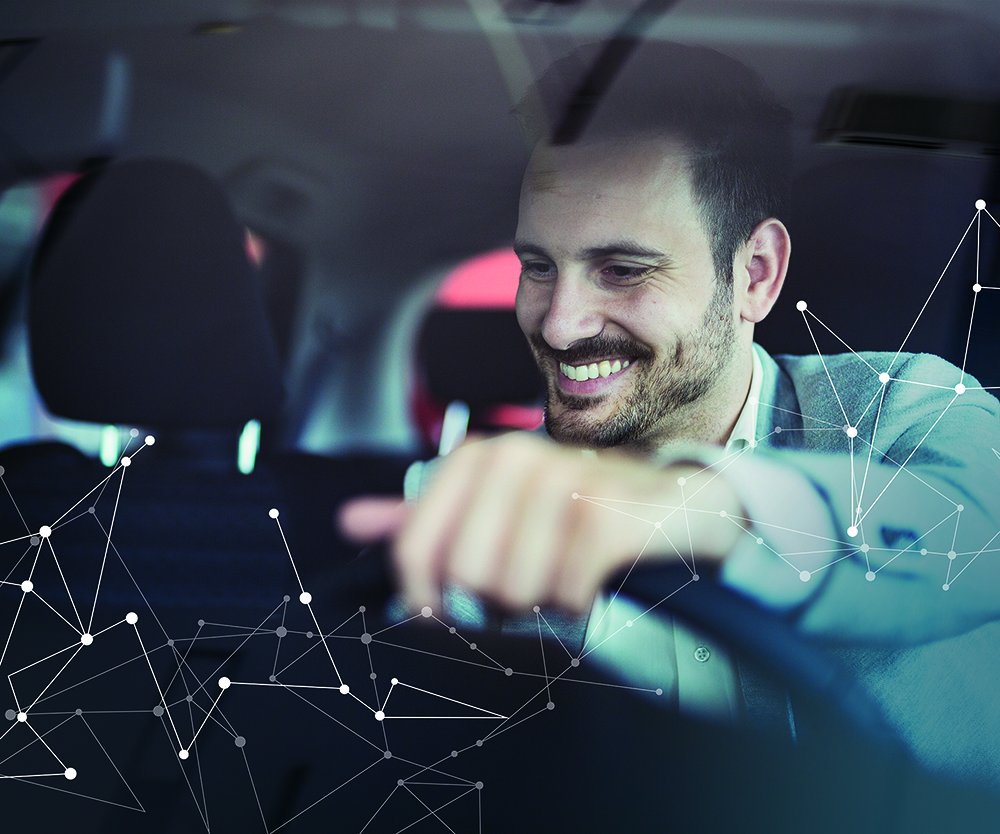
In many application areas, a detection of affective and cognitive states can be beneficial. For example, in areas such as usability testing, state detection can provide better insight into the effect of a product on the user and provide information about their possible overload with the product.
However, some states are expressed extremely subtly, making their detection a major challenge. For example, one modality, e.g. video, is not sufficient to robustly detect cognitive overload. This can only be made possible by combining different modalities, such as gaze detection and different biosignals.
The requirements and modalities may change depending on the deployment scenario. Also, interfering factors can affect the signals and create uncertainties in the detection of the condition.
The goal of this application is therefore to develop a modular and robust system for multimodal state detection. For this purpose, in addition to data fusion, the quantification of uncertainties in particular plays an important role, enabling an assessment of the reliability of individual modalities in order to incorporate them accordingly into the overall assessment.