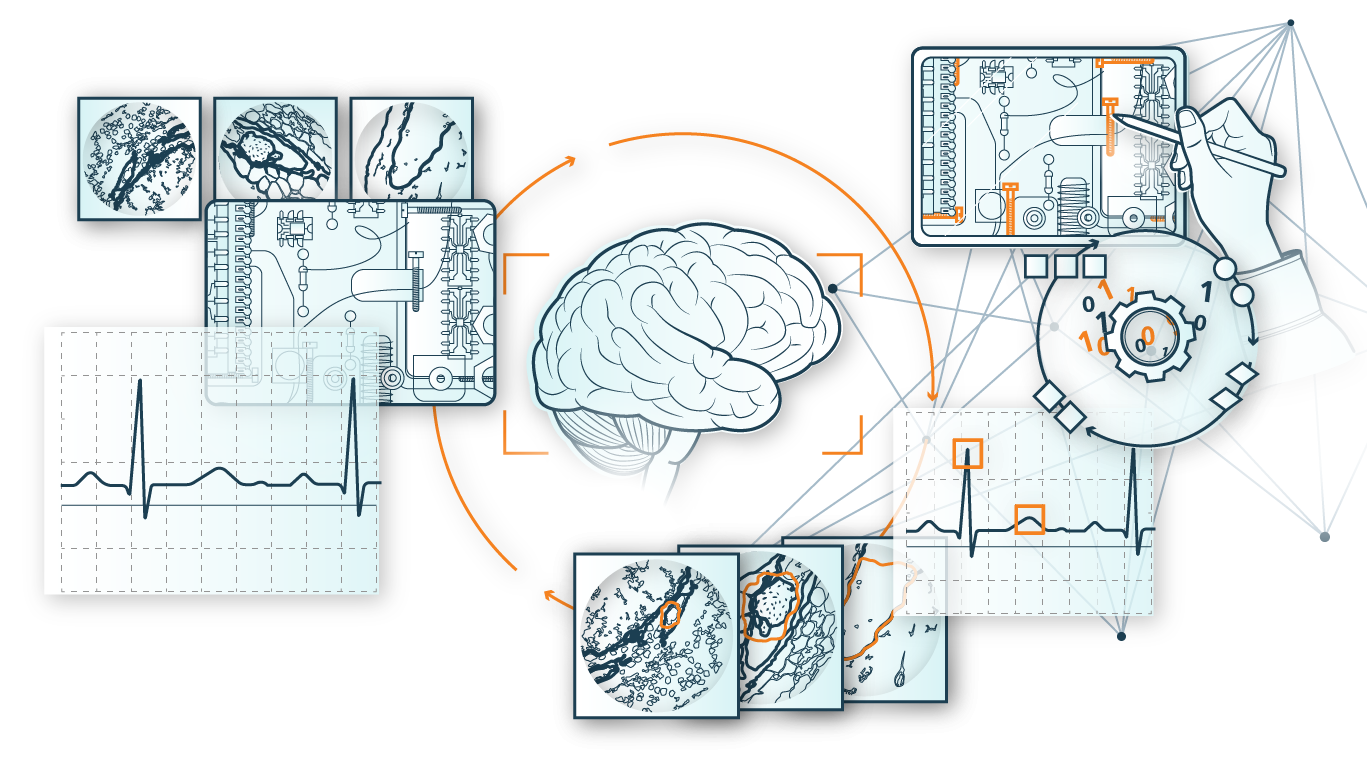
Data is the raw material for all machine learning and artificial intelligence applications. Useful and meaningful insights based on this data can only be extracted if the knowledge associated with or contained in it, i.e. its »semantics«, is captured in a suitable way during or after the creation of the data, described in a suitable form, i.e. equally in a representation understandable by humans and machines, and correlated with the actual data.
With reference to these requirements, the competence pillar »Semantics« deals with two key areas:
- Acquisition of knowledge: The first key area focuses on the question of how »model knowledge« in various specific application areas (such as driver assistance, self-localization, digital pathology, or segmentation of XXL tomography data) can be captured and jointly described with the measurement data used and required for this purpose (e.g., vital data and emotions of persons in the vehicle, localization parameters, microscopy data of histological tissue, XXL tomography data).
- The second key area deals with the challenge of linking the captured information or semantics with the associated measurement data in such a way that these can be made available and usable for various applications by means of methodological approaches from the fields of data analysis, machine learning and artificial intelligence.