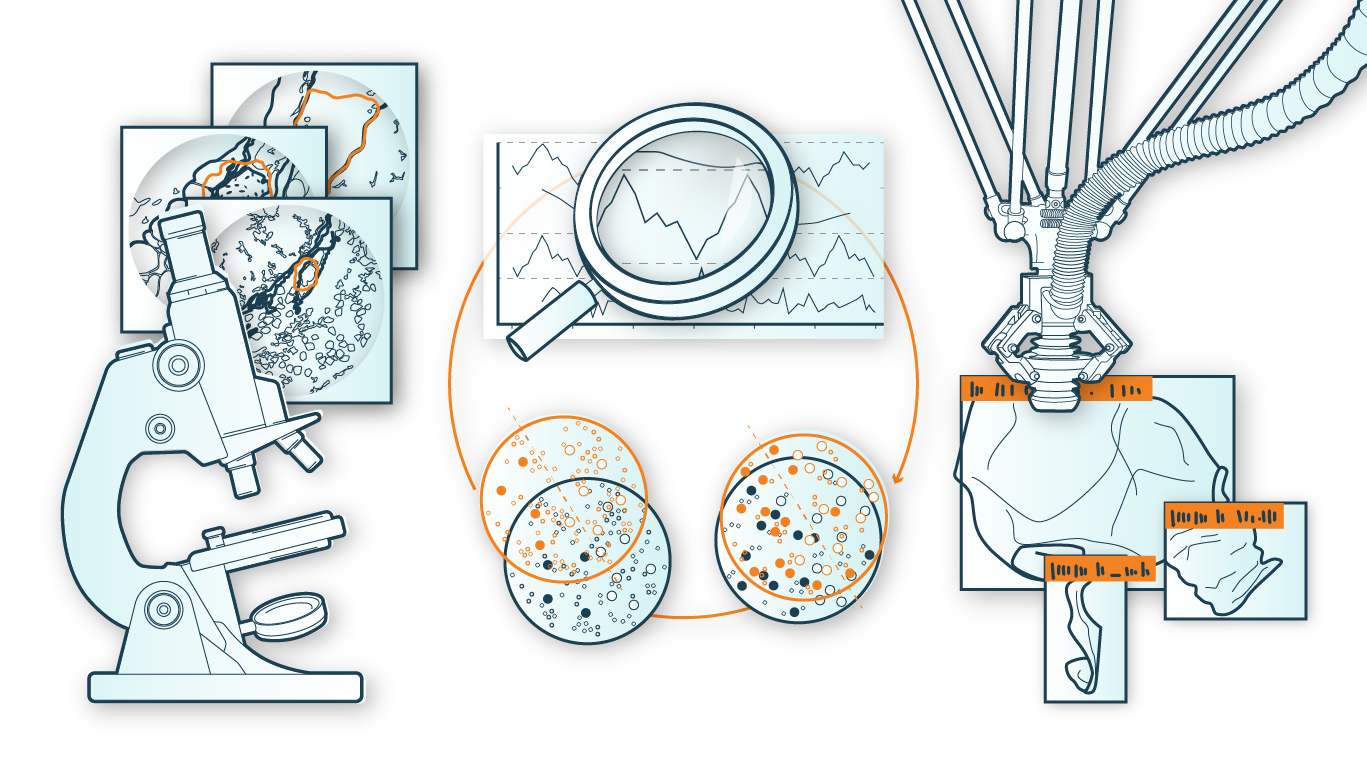
The groundbreaking successes of artificial intelligence (AI) in tasks such as speech recognition, object recognition, and machine translation are due in part to the availability of enormously large annotated data sets. Annotated data, also called labeled data, contains the label information that makes up the meaning of individual data points and is essential for training machine learning models. In many real-world scenarios, especially in industrial environments, large amounts of data are often available, but they are not annotated or only poorly annotated. This lack of annotated training data is one of the major obstacles to the broad application of AI methods in the industrial environment. Therefore, in the competence pillar »Few Labels Learning«, learning with few annotated data is explored within three focus areas and different domains: meta-learning strategies, semi-supervised learning, and data synthesis.